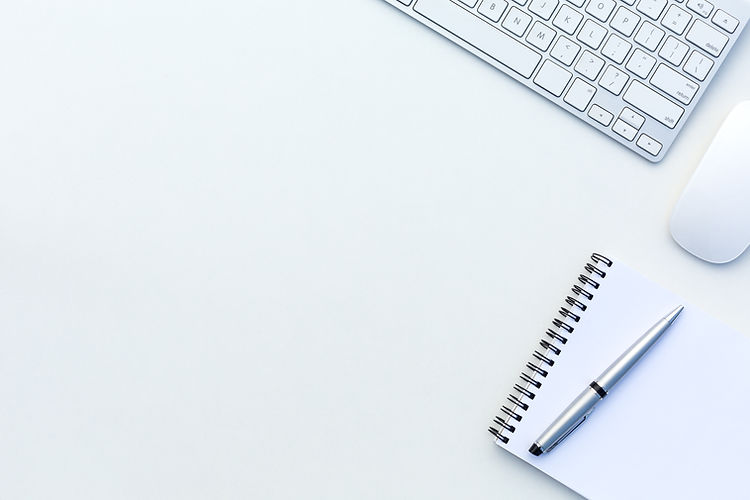

Spatially resolved transcriptomics provides a new way to define spatial contexts and understand the pathogenesis of complex human diseases. Although some computational frameworks can characterize spatial context via various clustering methods, the detailed spatial architectures and functional zonation often cannot be revealed and localized due to the limited capacities of associating spatial information. We present RESEPT, a deep-learning framework for characterizing and visualizing tissue architecture from spatially resolved transcriptomics. Given inputs such as gene expression or RNA velocity, RESEPT learns a three-dimensional embedding with a spatial retained graph neural network from spatial transcriptomics. The embedding is then visualized by mapping into color channels in an RGB image and segmented with a supervised convolutional neural network model. Based on a benchmark of 10x Genomics Visium spatial transcriptomics datasets on the human and mouse cortex, RESEPT infers and visualizes the tissue architecture accurately. It is noteworthy that, for the in-house AD samples, RESEPT can localize cortex layers and cell types based on pre-defined region- or cell-type-enriched genes and furthermore provide critical insights into the identification of amyloid-beta plaques in Alzheimer's disease. Interestingly, in a glioblastoma sample analysis, RESEPT distinguishes tumor-enriched, non-tumor, and regions of neuropil with infiltrating tumor cells in support of clinical and prognostic cancer applications.
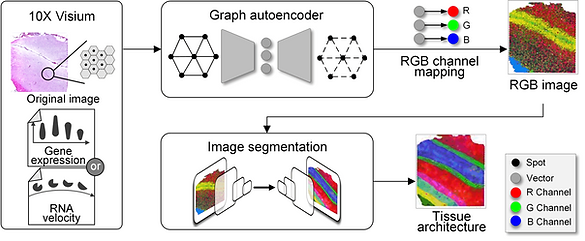

The tissue module (TM) was defined as an architectural area containing recurrent cellular communities executing specific biological functions at different tissue sites. However, the computational identification of TMs poses challenges owing to their various length scales, convoluted biological processes, not well-defined molecular features, and irregular spatial patterns. Here, we present a hypothesis-free graph Fourier transform model, SpaGFT, to characterize TMs. For the first time, SpaGFT transforms complex gene expression patterns into simple, but informative signals, leading to the accurate identification of spatially variable genes (SVGs) at a fast computational speed. Based on clustering the transformed signals of the SVGs, SpaGFT provides a novel computational framework for TM characterization. Three case studies were used to illustrate TM identities, the biological processes of convoluted TMs in the lymph node, and conserved TMs across multiple samples constituting the complex organ. The superior accuracy, scalability, and interpretability of SpaGFT indicate that it is a novel and powerful tool for the investigation of TMs to gain new insights into a variety of biological questions.
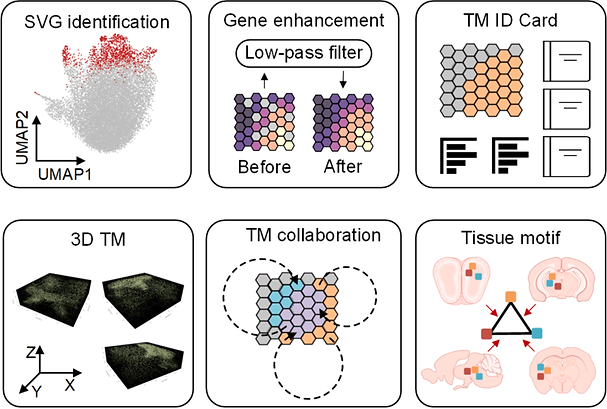